»It is hard to imagine a person who would feel comfortable in blindly agreeing with a
system’s decision without a deep
understanding of the decision-making rationale.―
Detailed explanations of AI decisions seem necessary to provide insight into the rationale the AI uses to draw a conclusion.«
»Only when users and stakeholders understand how and what predictions AI systems arrive at these systems can be used responsibly to make important decisions.«
Welcome!
This site is dedicated to my research on explainable artificial intelligence, driven by the vision that machine learning models should not only make decisions or provide probabilities, but also provide human-understandable explanations for how they reach their conclusions.
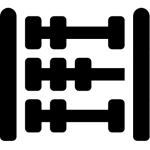
Accuracy
Machine learning models must be accurate to be able to provide reasonable explanations.
We validate all models in independent datasets to test their performance.
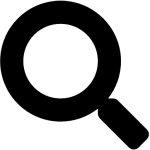
Focus
Machine learning models need to focus on key features that are causally related to the target and often known a priori.
Relevance maps help us to assess the level of noise and to detect bias in the training data.
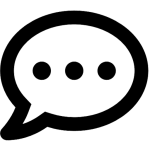
Explanation
Machine learning models should provide an explanation describing the decision-making process in an intuitive way.
Our goal is to develop a system architecture that can extract relevant information and dynamically synthesize descriptive explanations.
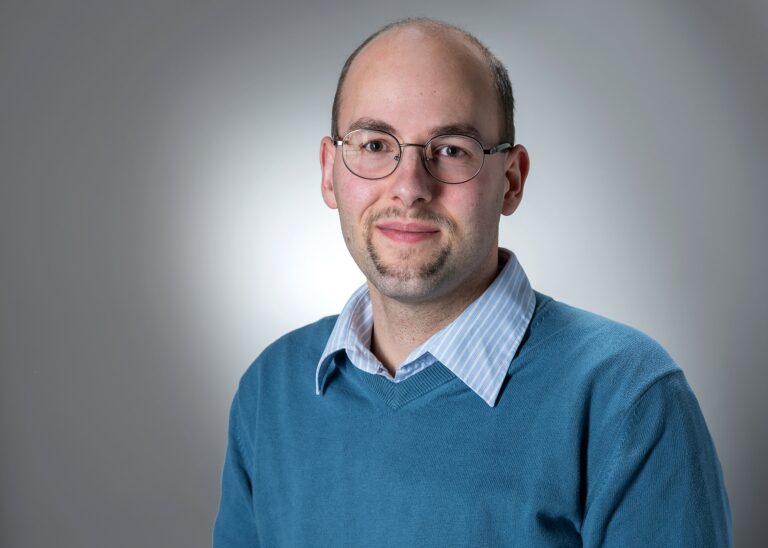
Martin Dyrba, PhD
I am a researcher in artificial intelligence and translational research in neuroimaging. My research interests are machine learning methods to detect neurodegenerative diseases such as Alzheimer’s.
At present, I am research associate at the German Center for Neurodegenerative Diseases (DZNE), Rostock. Here, I work on novel approaches to improve the comprehensibility and interpretability of machine learning models.
Biosketch & activities
In 2011, I graduated from the University of Rostock. In 2016, I obtained my PhD in medical informatics. At the end of 2015, I was awarded the Steinberg-Krupp Alzheimer’s Research Prize for my work on Support Vector Machine models to detect Alzheimer’s disease based on multicenter neuroimaging data. I have been working as reviewer for several grant agencies and international journals. I was guest editor for the Frontiers Research Topic ‘Deep Learning in Aging Neuroscience’
Recently, I chaired the Featured Research Sessions ‘Doctor AI: Making computers explain their decisions’ at the Alzheimer’s Association International Conference (AAIC) 2020 and the annual meeting of the German Association for Psychiatry, Psychotherapy and Psychosomatics (DGPPN) 2021.
In 2020, I developed a convolutional neural network architecture to detect Alzheimer’s disease in MRI scans. The diagnostic performance was validated in three independent cohorts.
From the neural networks, we can derive relevance maps that indicate the brain areas with high contribution to the diagnostic decision. Medial temporal lobe atrophy was shown as most relevant area which matched our expectations, as hippocampus volume is actually the best established neuroimaging marker for Alzheimer’s disease.
We are working on a system architecture that can extract relevant information and dynamically generate descriptive explanations of varying granularity on demand.
“AI will drastically change healthcare. We are working on making AI systems more reliable, transparent, and comprehensible.”
Team members
Current members
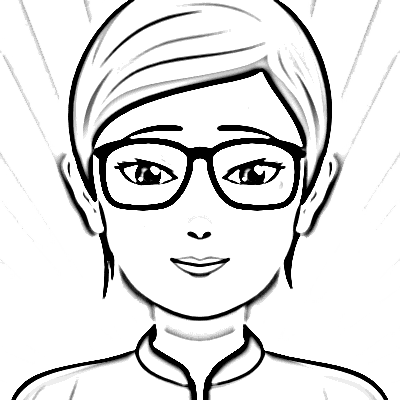
This could be You
We are constantly looking for talented student assistants to support our team. Please contact us.
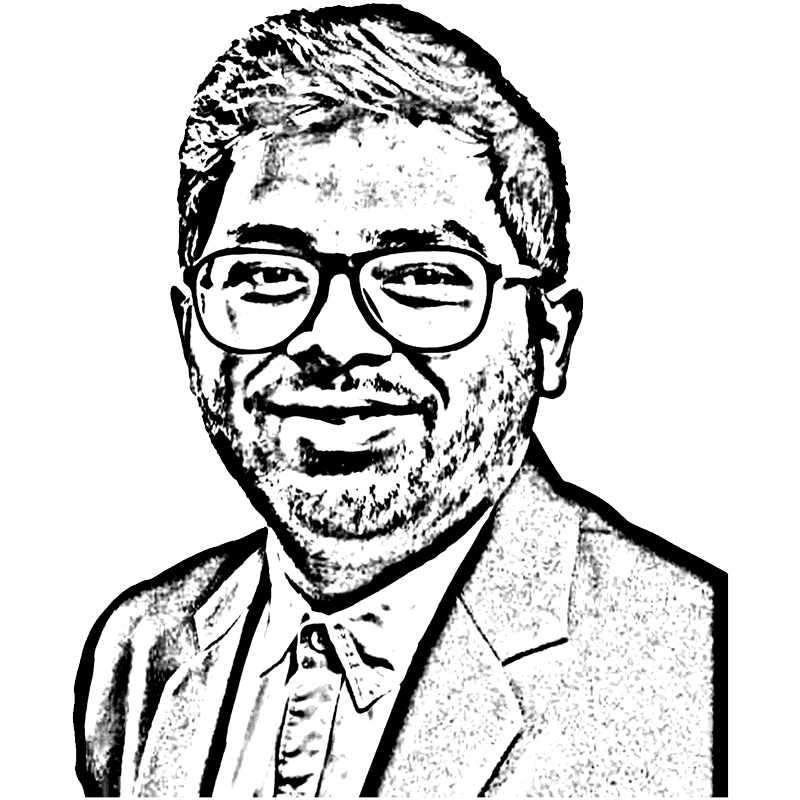
Devesh Singh, MSc
PhD student, DFG project:
Encoding semantic knowledge in CNNs for generating textual explanations
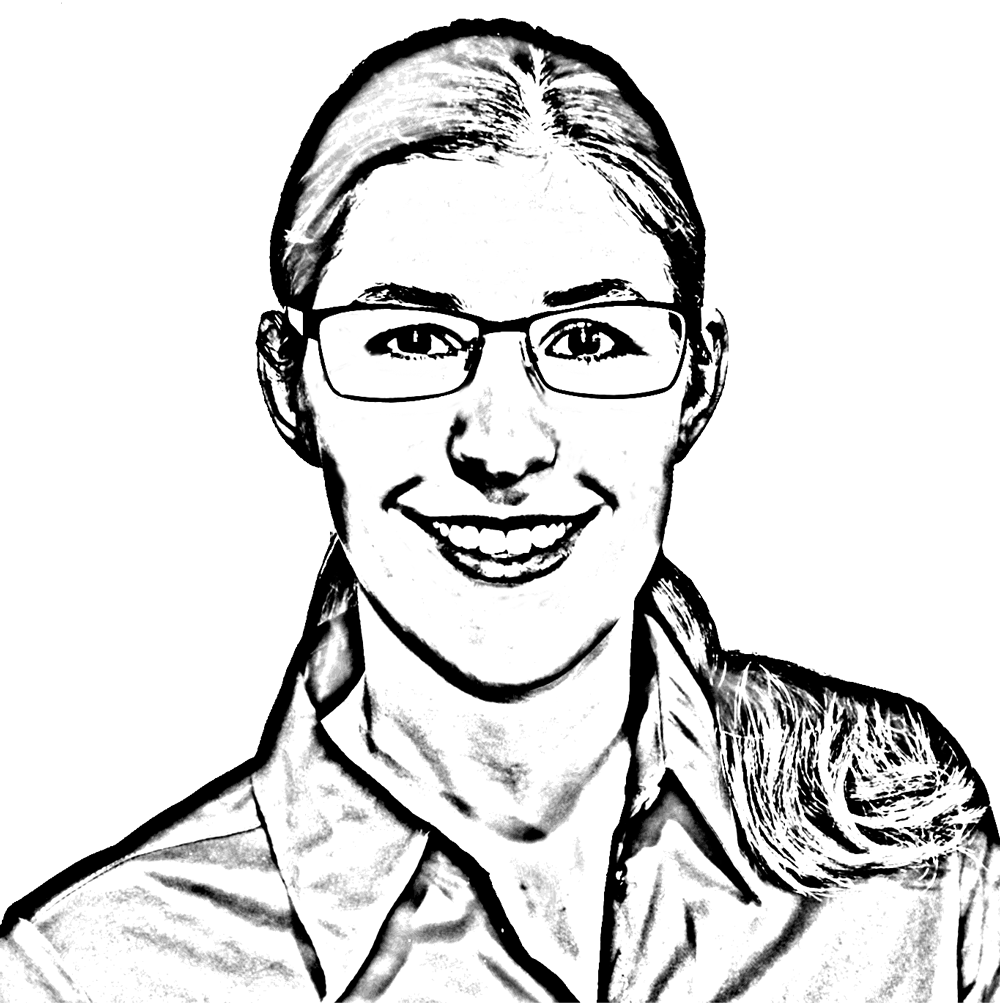
Doreen Görß, MD
Neurologist and Postdoc,
EU Interreg BSR project:
Clinical AI-based Diagnostics (CAIDX)
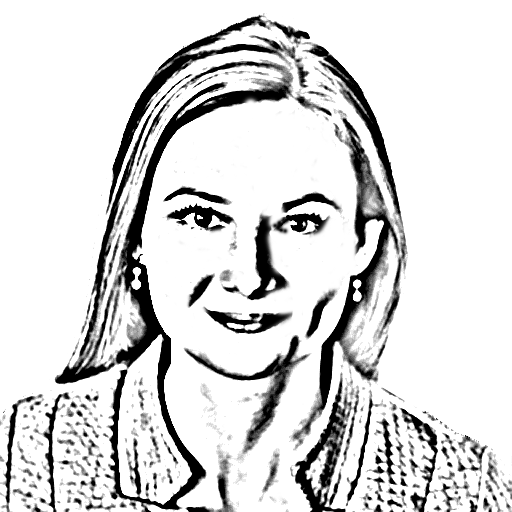
Olga Klein, PhD
Psychologist and Postdoc,
BMBF project:
Theoretical, Ethical and Social Implications of AI-based Computational Psychiatry (TESIComp)
Sarah Wenzel
Student assistant, since 2021
Survey & interview design and analysis
Aminah Dodiya
Master student
Federated ensemble learning for the detection of Alzheimer's disease in MRI scans
▷ Alumni - Former members
▷ Alumni - Former members
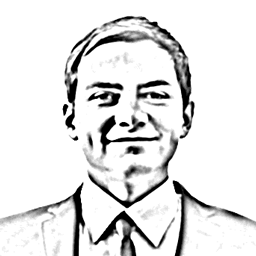
Vadym Gryshchuk
PhD candidate, 2021-2022
Detection of frontemporal dementia using contrastive self-supervised learning
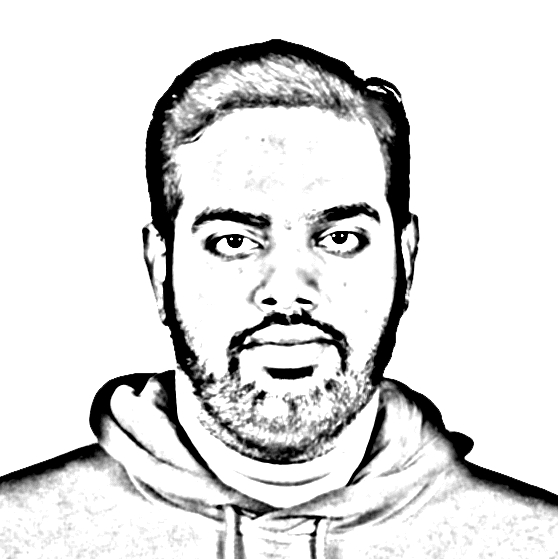
Zain Ul Haq
Master's Thesis, 2022
Detection of frontotemporal dementia by learning with fewer training samples
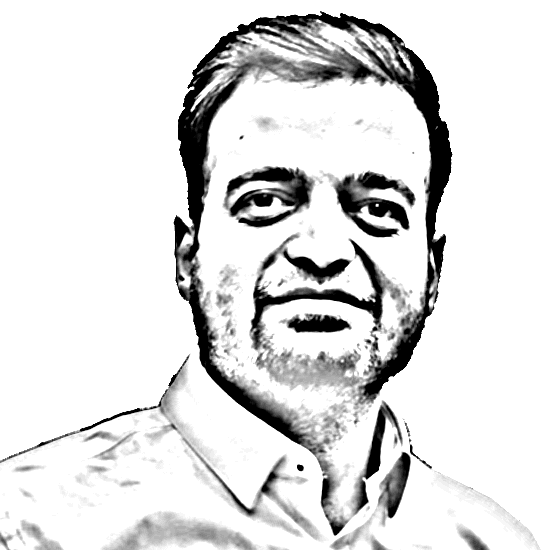
Muhammad Usman
Master's Thesis, 2022
Learning visual representations from 3D brain images using self-supervised techniques
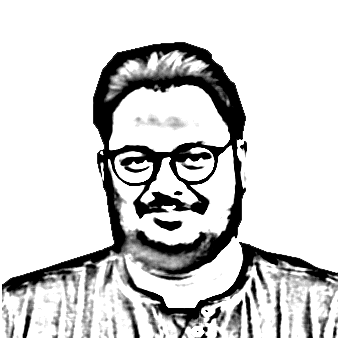
Shabbir Ahmed Shuvo
Master's Thesis, 2022
Application of BYOL Self-Supervised Learning (SSL) on MRI data in case of Alzheimer's disease
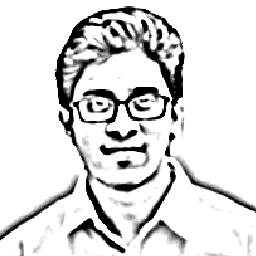
Md Motiur Rahman Sagar
Master's Thesis, 2020
Learning shape features and abstractions in convolutional neural networks
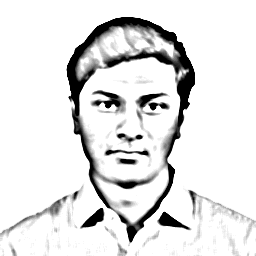
Arjun Haridas Pallath
Master's Thesis, 2020
Comparison of convolutional neural network training parameters
Moritz Hanzig
Student assistant, 2020
InteractiveVis Python and Bokeh programming
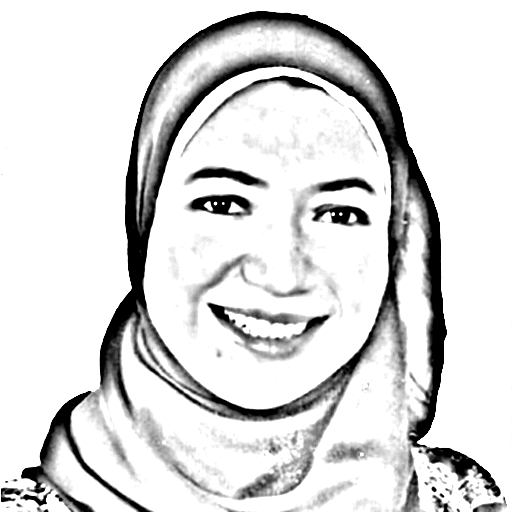
Eman N. Marzban
Guest researcher from Cairo, Egypt, 2018
Visualization methods for convolutional neural networks
Contact
Contact
Martin Dyrba
Phone
+49.381.494.9482
martin.dyrba (at) dzne.de
Location
Rostock, Germany